Distributed Dictionary
The Dragon Distributed Dictionary implements a data distributed key-value store that spans multiple nodes of a distributed program’s allocation. This makes it possible to create large key-value stores, larger than could be stored in memory on one node. It also distributes access to the key-value store so that there is no bottlekneck in accessing or storing values. Access to the store is distributed according to an evenly distributed hashing algorithm, thus statististically spreading the data evenly over all the nodes of the store.
The next section describes the design of the Distributed Dictionary followed by Performance Tips which offers some tips and pointers to things to read on how to get the best performance from the Distributed Dictionary in your application.
Design
A design requirement is for the distributed dictionary to scale to 10,000 managers and 100,000 clients. Any centralized focal point is going to be a bottleneck for a design that must scale to these sizes. The design presented here reflects that need by minimizing the overhead of clients attaching and detaching from the distributed dictionary.
To implement the key-value store there are three entities that are named:
Clients access the key-value store primarily using get and put operations though other operations are also defined.
An Orchestrator provides coordination services and orchestrates startup, teardown, and client attaching and detaching activities.
Managers provide access to data that is stored within their shard of the key-value store, generally handling the interaction between clients and data stored within a manager’s shard.
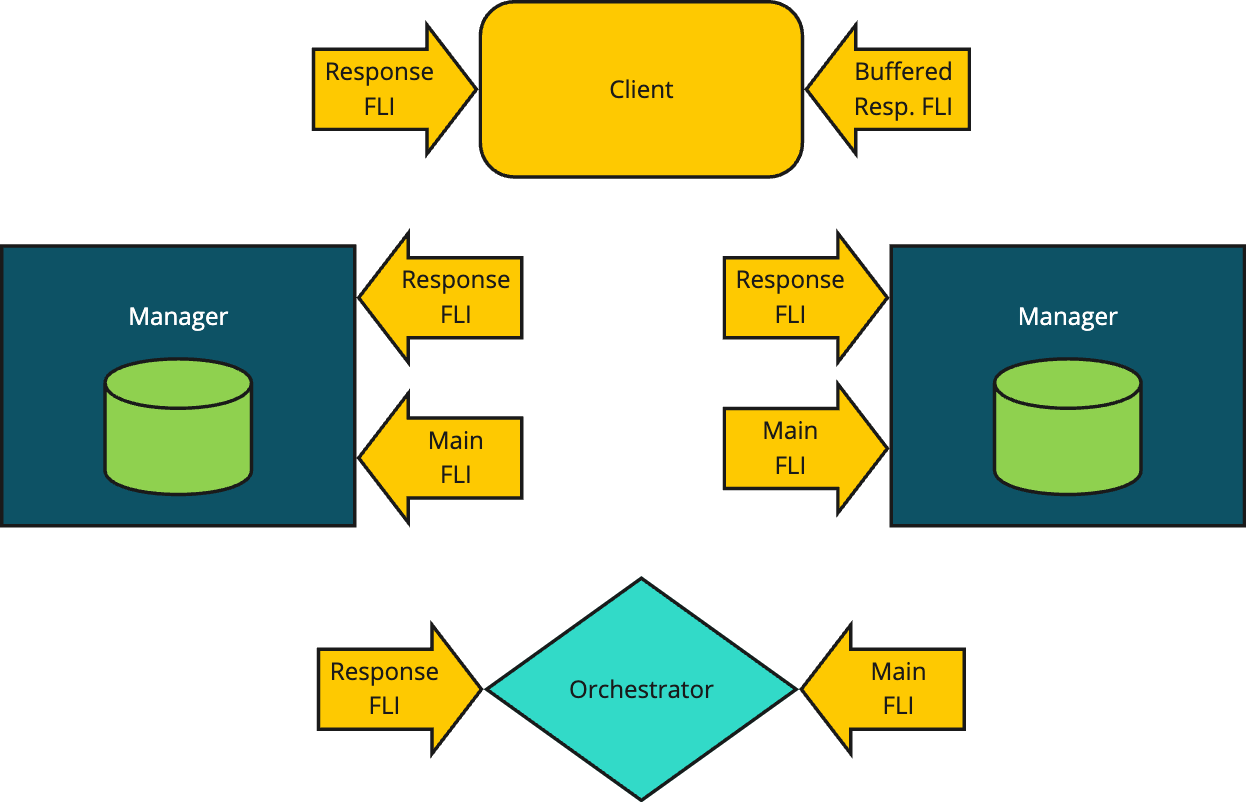
Fig. 25 Conceptual View of a Distributed Dictionary
Clients connect with managers based on the hashed values of the keys they are either looking up or the key-value pairs they are storing. The orchestrator starts the managers and is responsible for negotiating the connections between clients and managers. The orchestrator is also responsible for tearing down a distributed dictionary. The following sections cover the interactions between the components in detail depicting the messaging that is exchanged between them during lifetime management and operation of the key-value store.
1class MyKey:
2 import cloudpickle
3
4 def __init__(self, key_value):
5 self._key_value = key_value
6
7 def __hash__(self):
8 # hash_fun can be user-defined in whatever way
9 # makes the best selection. It might, for instance,
10 # return the index of the manager you wish to target.
11 return hash_fun(self._key_value)
12
13 def __getstate__(self):
14 return cloudpickle.dumps(self._key_value)
Normally the key value is hashed using a simple byte hashing algorithm which is callable from C, C++, and Python. From Python the key is serialized first, then a hash value is determined which finally determines which manager will hold a stored key-value pair. The distribution of that byte level hashing function is relied on to distribute the data amongst the managers in a statistically even manner.
Some applications may wish to have finer control over the placement of data in the distributed dictionary. For instance, in some applications it might be that data could be placed on the same node as clients which will write and read the data. In cases like this it is possible to define a class with its own hash function which can be used to determine which manager is selected. Once selected, the manager itself will still use the low-level byte hashing function itself, but selection of a manager can be determined by provding an appropriate class with its own hash function as shown in Listing 54. The getstate function is called when the key is serialized and the definition provided in the sample code simply strips off the extra MyKey definition and results in serializing the original key value.
Prominently in the Fig. 25 are the use of FLIs (i.e. File Like Interfaces). FLIs enable safe 1:1 conversations between a sender and a receiver. FLIs are configurable to allow safe 1:1 streaming conversations as well where the sender may stream content to a receiver over multiple messages. FLIs and streaming FLIs are used extensively in the design of the distributed dictionary and some familiarity with the FLI API is assumed. Each component has two FLIs as shown in the figure. In general a main FLI is used to service new incoming requests and a response FLI is used to handle responses to requests made by a component. In the case of a client, two response FLIs are created to handle streaming and non-streaming responses.
Every FLI is created of some set of channels. Each component within the distributed dictionary is responsible for creating its own FLIs and channels. The required channels are created using a new API, supported by Dragon Local Services on the node where a component is located. The new API, a class method named make_process_local is callable via the Channels API.
When a distributed dictionary is created by a client, the API first creates an Orchestrator which is told details about the size and configuration of the distributed dictionary being created. The orchestrator starts by creating two FLI interfaces, its main FLI and its response FLI. It publishes its main FLI to the client that created it by printing the serialized descriptor to the FLI to standard out of the Orchestrator.
The Orchestrator creates the specified number of Managers, providing each with the orchestrator response FLI and its main FLI. The orchestrator receives registration requests by each manager and responds to each manager on the manager’s resposne FLI.
Performance Considerations
Because of the way the Distributed Dictionary is designed, there are several knobs that can be used to optimize performance. Depending on your application and the system you are running on, you may get the best performance while using one or more of these optimizations.
On machines where there is more than on NIC (network interface card), you will likely obtain better performance by having more than one manager per node. This is because clients can access each manager completely in parallel when two NICs are present. When starting the dictionary you can specify the number of managers per node. If you benchmark your application you can play with this knob to see how it affects performance in your application. Even on machines without two NICs you may find that increasing the number of managers per node helps performance since each manager is doing work on its local shard of the dictionary and likely is not driving a single NIC to it maximum throughput.
In applications where data is purposefully sharded across multiple workers you can get much better performance if colocating workers with data is possible in your application. This is possible using the technique presented in Design. The idea is that you write a wrapper class for your keys as shown in Listing 54. The hash function would then return the manager id of the local manager. Consult the reference guide for the Python or C interface to see how to get the local manager’s id.
Checkpointing, covered in Checkpointing, is an effective way to use the dictionary to store generations of data and allow workers to be working with different generations. Unlike a database where there is only one version of data at any point in time, the Distributed Dictionary allows you to store more than one version corresponding to a checkpoint. What’s more, clients can work with different checkpoints at the same time which increases the amount of parallelism provided by workers since they don’t have to all be in lock step with each other. There are limits on the number of checkpoints that can be active at any point in time. This is called the working set size. If your code could benefit from checkpointing, playing with the working set size is a knob you can turn to tune performance.
Checkpointing
When using a distributed dictionary it may be desirable to checkpoint operations within the dictionary. Checkpointing is designed to minimize the interaction between clients and managers to allow for scaling the dictionary to the sizes needed to support large-scale supercomputing.
In addition to checkpointing computation, persisting a checkpoint to disk is also introduced, which is likely needed at scale. This is covered in more detail in the next section.
To implement checkpointing we want to avoid a global synchronization point to achieve the maximum amount of parallelization and minimum impact to processes. This means we need a protocol that allows some processes to work with data from a new checkpoint while others continue to work with an older copy. Inherent in this design is that all processes work on data that changes over time and there are discrete moments where the data is updated.
To implement checkpointing for these kinds of applications we introduce the concept of a working set. A working set is a set of checkpoints that all reside in memory at a given moment of time.
The algorithm introduces a checkpoint id. This id starts at 0 when a distributed dictionary is created. It is a 64-bit unsigned which can wrap around (but probably won’t). When a client wants to checkpoint it will invoke its checkpoint function which will simply increment the checkpoint id modulo the maximum 64-bit value (it will automatically wrap in C/C++, but will be added mod the max 64-bit size in Python). No interaction with the managers or orchestrator is necessary when a client checkpoints.
Another goal of checkpointing is to keep from copying data unnecessarily. All distributed dictionary client operations will include their checkpoint id. Creation, Read, and Write operations will proceed as follows in the subheadings below.
When a checkpointing distributed dictionary is created, it may be told to persist for every persist_freq number of checkpoints. When persisted, an argument, persist_base will provide the base filename. A unique filename for the checkpoint id and the particular manager id is constructed and used when persisting a checkpoint.
Example
Here is sample code for computing \(\pi\) which makes use of, and demonstrates, distributed dictionary checkpointing. The complete code is available at example/dragon_data/ddict/ddict_checkpoint_pi.py. In this program, we generate a set of random points within a square with a side length of 2. There is a circle inside the square with a radius of 1. The square and circle are centered at the origin of the coordinate system. We calculate the approximation of \(\pi/4`\) by the ratio of points that fall within the circle to the total number of points generated inside the square.
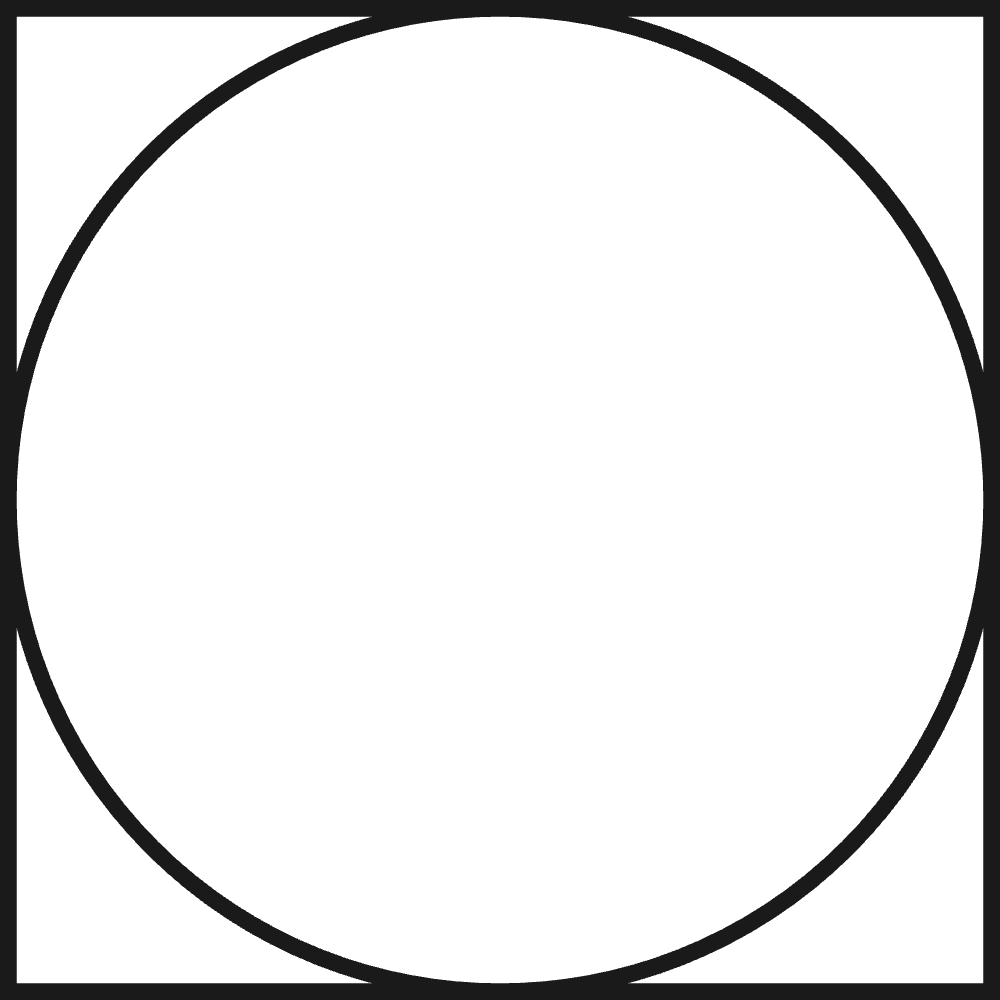
Fig. 26 The Unit Circle Inscribed in a Square
The derivation of the approach for approximating \(\pi\) focuses on the area of a circle vs the area of an enclosing square as depicted in Fig. 26. The square has area 4 which is computed from the side length of 2 which is the radius of the circle times 2 (i.e. the unit circle in this instance). For the square, \(Area_{square} =(2r)^2 = 4r^2\). The area of a circle is \(Area_{circle} =\pi r^2\). Counting random points and whether they land inside the circle, we approximate the probability of landing inside the circle. Every point generated lands inside the square so those that also land inside the circle gives us the ratio of points that land inside the circle over those that land inside the square (i.e. all points). The formula for the ratio is \((\pi r^2 / (4r^2)) = \pi / 4\). In our example, \(r=1\) but we are computing a ratio so it would work with any \(r\). It takes a lot of points to accurately compute \(\pi\). The algorithm provided here computes it to the point where it is converging slower than the number of decimal places provided.
1import dragon
2import multiprocessing as mp
3import argparse
4import traceback
5import math
6import numpy as np
7
8from dragon.data.ddict import DDict
9from dragon.native.machine import System
10
11# The number of worker/clients of the distributed dictionary.
12NUM_PROCS = 128
13
14
15def in_circle(point):
16 x = point[0]
17 y = point[1]
18 return math.sqrt(x**2 + y**2) <= 1
19
20
21def proc_function(d: DDict, client_id: int, places: float, trace: bool) -> None:
22 """Calculate an approximation of PI
23
24 :param d: distributed dictionary
25 :type d: DDict
26 :param client_id: client ID of the process
27 :type client_id: int
28 :param places: Stops the simulation if the answer has converged to less than this amount of change.
29 :type places: float
30 :param trace: set to True to print out the result in each iteration
31 :type trace: bool
32 """
33 try:
34 d.pput(f"result{client_id}", 0)
35 # Not necessary here to access num_procs via dictionary, but demonstrates persistent keys.
36 num_procs = d["num_procs"]
37 num_points = d["num_points"]
38 step = 0
39 avg = 0
40 done = False
41 while not done:
42 # generate a set of random points in each iteration
43 rand_points = np.random.uniform(-1, 1, (num_points, 2))
44 num_points_in_circle = 0
45 for point in rand_points:
46 if in_circle(point):
47 num_points_in_circle += 1
48
49 # calculate the ratio of points fall within the circle to total number of points generated
50 new_sim = num_points_in_circle / num_points
51
52 # calculate the weighted average of the ratio
53 local_avg = ((num_procs * step * num_points) * avg + new_sim * num_points) / (
54 (num_procs * step * num_points) + num_points
55 )
56
57 # write new simulation result to dictionary as a non-persistent key that will be updated in every future checkpoint
58 d[client_id] = local_avg
59 if trace:
60 print(f"Checkpoint {step}, client {client_id}, local average is {local_avg*4}", flush=True)
61
62 # calculate the average of the result from all other clients for this checkpoint
63 sum_of_avgs = 0
64 for i in range(num_procs):
65 # This blocks until average is available from other clients for this checkpoint
66 sum_of_avgs += d[i]
67 prev_avg = avg
68 avg = sum_of_avgs / num_procs
69
70 # stop the loop if the it has converged to a value that changes less than places
71 # on each iteration.
72 if abs(avg - prev_avg) < places:
73 done = True
74
75 # moving to the next checkpoint/iteration
76 d.checkpoint()
77 step += 1
78
79 print(f"PI simulation result = {4*avg} from client {client_id}.", flush=True)
80 d.detach()
81 print(f"Client {client_id} now detached from dictionary.", flush=True)
82
83 return local_avg
84
85 except Exception as ex:
86 tb = traceback.format_exc()
87 print(f"caught exception {ex}\n{tb=}", flush=True)
88
89
90def main():
91 mp.set_start_method("dragon")
92 parser = argparse.ArgumentParser(description="Distributed Dictionary Training Example")
93 parser.add_argument(
94 "--digits", type=int, default=10, help="Number of places after the decimal point of PI accuracy"
95 )
96 parser.add_argument(
97 "--trace",
98 nargs="?",
99 type=bool,
100 default=False,
101 const=True,
102 help="Set to True to print simulation result for all clients in each iteration",
103 )
104
105 my_args = parser.parse_args()
106 places = 5 ** -(my_args.digits + 1)
107
108 my_alloc = System()
109 nnodes = my_alloc.nnodes
110 d = DDict(1, nnodes, nnodes * int(4 * 1024 * 1024 * 1024), wait_for_keys=True, working_set_size=4, timeout=200)
111 procs = []
112
113 # write meta data to dictionary as persistent keys
114 d.pput("num_procs", NUM_PROCS)
115 d.pput("num_points", 100000) # each client generate 100000 points in each iteration
116
117 fun_args = [(d, i, places, my_args.trace) for i in range(NUM_PROCS)]
118
119 with mp.Pool(processes=NUM_PROCS) as pool:
120 results = pool.starmap(proc_function, fun_args)
121
122 # sync up head process to the newest checkpoint - not needed, but demonstrates
123 d.sync_to_newest_checkpoint()
124
125 print(f"Globally there were {d.checkpoint_id+1} checkpoints that were performed.", flush=True)
126
127 # calculate the average result from final iteration
128 # This would be one way to do it. In this case we have the results from the map function.
129 # sum_of_avgs = 0
130 # for i in range(NUM_PROCS):
131 # # This blocks until average is available from other clients for this checkpoint
132 # sum_of_avgs += d[i]
133 avg = sum(results) / NUM_PROCS
134
135 print(f"The result is {round(4*avg, my_args.digits)}", flush=True)
136 print(f"Stats of ddict: {d.stats}", flush=True)
137
138 d.destroy()
139
140
141if __name__ == "__main__":
142 main()
The code in Listing 55 makes use of a distributed dictionary with a working set size of 4. This means that up to 4 checkpoints can exist in each manager of the distributed dictionary. In addition, the wait_for_keys being specified means that clients, i.e. the proc_function, will remain synchronized as they progress through their iterations since each client depends on the results of the other clients as it computes.
The use of the multiprocessing pool is a scalable means of creating the clients of this simulation. Each instance of the proc_function will compute and return its local average value. As seen in Listing 55, an equivalent method would have been to retrieve the result from the distributed dictionary, but for this final result in this program, collecting the results via the starmap multiprocessing map function is more efficient.
(_env) .../hpc-pe-dragon-dragon/examples/dragon_data/ddict $ dragon ddict_checkpoint_pi.py --trace
chkpt 0, client 1 local_avg=0.78423
chkpt 0, client 0 local_avg=0.78617
...
chkpt 1, client 0 local_avg=0.7857033333333333
chkpt 1, client 2 local_avg=0.7857378787878788
chkpt 1, client 5 local_avg=0.785789393939394
...
chkpt 39, client 9 local_avg=0.7856719183793902
chkpt 39, client 10 local_avg=0.785670221021504
chkpt 39, client 28 local_avg=0.7856690040479251
pi simulation result = 3.1426821260796105
...
Globally there were 40 checkpoints that were performed.
The result is 3.1426821261
Stats of ddict: [DDictManagerStats(manager_id=0, total_bytes=2147483648, total_used_bytes=745472, bytes_for_dict=2147483648, dict_used_bytes=745472, overhead_used_bytes=0, num_keys=91), DDictManagerStats(manager_id=1, total_bytes=2147483648, total_used_bytes=581632, bytes_for_dict=2147483648, dict_used_bytes=581632, overhead_used_bytes=0, num_keys=71)]
+++ head proc exited, code 0
Running this demo program as shown in the abbreviated output of Listing 56 shows that the thirty-nine checkpoints where generated globally in the application (i.e. across the two managers) and the data was distributed as shown in the output with 91 keys stored at manager 0 and 71 keys stored at manager 1. The trace option displays output as the clients run helping to illustrate what the code is doing.
Dictionary Creation
Internally to each manager, one dictionary per checkpoint copy in the working set is maintained. For our purposes we’ll call each working set item a checkpoint. A checkpoint is a local, internal Python dictionary inside the manager. A manager manages one shard of the distributed data and one checkpoint corresponds to one internal dictionary that keeps track of the key/value pairs that were modified during that checkpoint lifetime.
When a dictionary is created, one pool is created which is big enough to hold all the working set of the distributed dictionary. By doing this, no copying of values is necessary between working sets. Working sets are maintained entirely by the internal manager dictionaries that manage the keys and values.
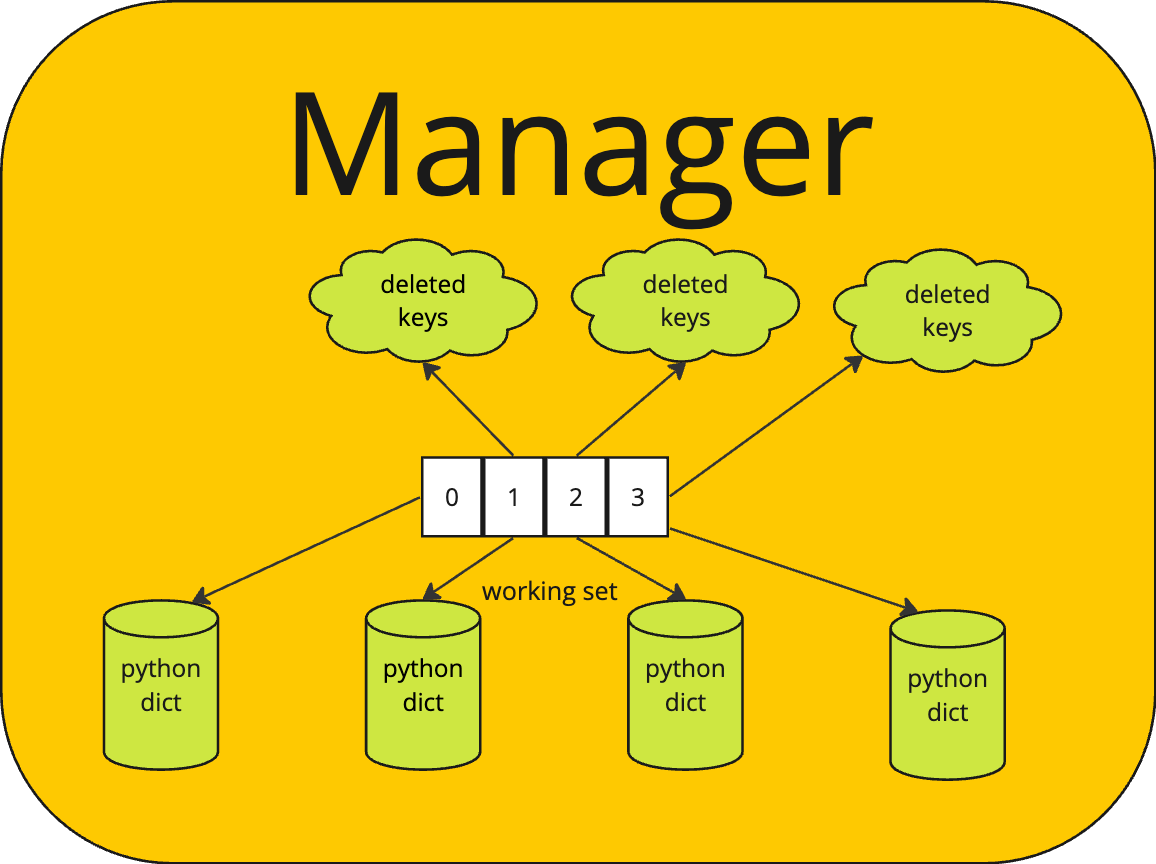
Fig. 27 Detailed View of Manager
The internal dictionaries are maintained in a map of the working set, called working_set as pictured in Fig. 27. A map (i.e. another internal dictionary) called working_set, maps the checkpoint number to a tuple of the deleted keys and the checkpoint’s working dictionary. Initially the checkpoint id is 0 for all clients and each dictionary in the working_set of each manager is empty. The working set contains dictionaries for 0, 1, 2, on up to the working_set_size to begin.
For each working set member (i.e. checkpoint), except the oldest, there is a deleted_keys set. This is a set of all keys that were deleted for a given checkpoint id. If a new value is stored in the working set for a checkpoint id level, then the key is removed from the deleted_keys set. The deleted_keys set is added to when a key is deleted from a checkpoint, but it exists at an older checkpoint. Otherwise, the key is just deleted from the checkpoint in which it exists.
1d = DDict(managers_per_node=None, num_nodes=None, total_mem=None, working_set_size=1,
2 wait_for_keys=False, wait_for_writers=False, policy=None, persist_freq=None,
3 persist_base_name=None, timeout=None)
Listing 57 shows the signature for creating a distributed dictionary. When creating a distributed dictionary it is possible to specify the number of managers and number of nodes on which the distributed dictionary will be deployed. It is also possible to determine in more detail, for example on which nodes, a distributed dictionary will be deployed by providing a policy on the policy argument. The working set size may be specified as described above. A working set size of one means that no checkpointing will occur. A size of two or more allows checkpointing. Creating a working set size of more than two enables additional distributed parallelism by allowing clients to operate more independently.
In certain use cases it may be that there are a set of keys that should not persist between checkpoints AND that all keys written into one checkpoint should be written into all checkpoints. To get this behavior, the wait_for_keys argument should be set to True. In this case, it will be desirable to wait for all such keys to be written. In this case, keys that are written as d[key] = value will be assumed to be part of this set of keys. A second method of storing key/value pairs by writing d.pput(key, value) will result in writing a key/value that persists across checkpoints (i.e. persistent put) and will not be a part of the set of keys that are waited upon.
Waiting for keys means that a client that does a read or write for a checkpoint that currently does not exist in the working set (i.e. beyond the end of the working set) will block until all the keys in the retiring checkpoint have been written and all clients have moved on to new checkpoints.
With this mode of operation, readers of key/values in a checkpoint will also block if the key is not yet available. Once available, the reader will get the value and continue with execution.
All blocking operations are subject to a timeout. A timeout of None indicates to the distributed dictionary that clients want to wait forever for their requests to be satisfied. Specifying timeout values is application specific, but providing a timeout is a good idea. When creating a dictionary, the timeout that is specified is propagated to the orchestrator and through it to all managers as well providing a global timeout to the entire distributed dictionary and all operations that should be subject to a timeout. A default value of 10 seconds is provided, but this may be overridden by providing a timeout when the dictionary is constructed.
A less restrictive option is to set wait_for_writers to True when creating the distributed dictionary. In this case, all keys persist in the distributed dictionary across checkpoints, but all writers must have advanced their checkpoint before a checkpoint can be retired. It is assumed in this mode that writers that have written to the dictionary in the past will also be writing the same keys in the future. In this case then the distributed dictionary manager can monitor all writers and require that they have moved on from a checkpoint before retiring an older one.
Under the wait_for_writers requirements, a writer requesting to move on from a checkpoint will wait (i.e. block) if there are other writers that are still writing at a checkpoint that would be retired. If a reader moves on to new checkpoints, then it would continue, unblocked since keys persist across checkpoints and reader that are reading at a newer checkpoint can still see key/value pairs written in the past.
These subtle differences in blocking and distributed dictionary behavior should be carefully considered when writing an application. They provide different behavior and synchronization opportunities.
The persist_freq refers to a frequency that the distributed dictionary should be persisted. It will be persisted as checkpoints are retired. The frequency refers to how often a retiring checkpoint should be persisted. The persist_base_name is used to determine the name of the persisted state. The manager_id of the manager is appended to the base name followed by the checkpoint id of the persisted state.
Retiring and Persisting Checkpoints
A checkpoint that will no longer be in the working set is removed from the working set and retired. This is done with little to no impact on the processes that are using the dictionary. When the checkpoint is about to be retired a few checks are made depending on the options that were selected when the dictionary was created.
Checkpoints are retired when a client attempts to write into a new checkpoint that has not been set up yet. In that case, the oldest checkpoint is retired and a new checkpoint is created.
If wait_for_keys was chosen when the dictionary was created, then all non-persisting keys in the retiring checkpoint must exist in the next newer checkpoint. If they do not, then the request to move to a new checkpoint will be enqueued until later on an internal queue of requests to be processed later. Under this option, even reads of non-persisting keys will be queued if the newer checkpoint id does not exist. Any operations that attempt to get the state for a newer checkpoint that depends on the keys of the newer checkpoint will also be enqueued until the newer checkpoint exists.
If wait_for_keys was chosen when the dictionary was created, then the retiring checkpoint keys that are in the set of keys to persist are deep copied to the next newer checkpoint unless the next newer checkpoint has the key in its deleted_keys set or the key is already present in the newer checkpoint.
If wait_for_writers was chosen when the dictionary was created, then all writers into a checkpoint must have advanced to a checkpoint id greater than the one to be retired before the checkpoint can be retired. If all writers have not advanced, then the request to move to a new checkpoint will be queued internally until it can be processed.
If wait_for_keys was not specified on creation of the dictionary, then all keys are treated as persisting keys when checkpoints are retired.
If the retiring checkpoint is to be persisted, then all key/value pairs in the retiring checkpoint are written to persistent storage. The retiring checkpoint’s internal dictionary is handed over to a process to persist the values to disk. As it does so, the key/value pairs are deleted from the pool of the manager, thereby releasing those resources. The persisting of the data can occur completely independent of client interactions with the distributed dictionary. There are no shared data resources except the pool which is already multi-thread and multi-process safe. Otherwise there are no shared resources.
One possible design for persisting to disk is to form DDPut messages (or another capnp message) for each key/value pair in the pool and write them to a file descriptor which represents a file opened by the manager. The captain proto library supports writing to a file descriptor and we have a message already that contains the checkpoint number, the key, and the value. When recovery was initiated, a process could open the file, read the messages, and route the messages right into the manager to restore it to that point in time.
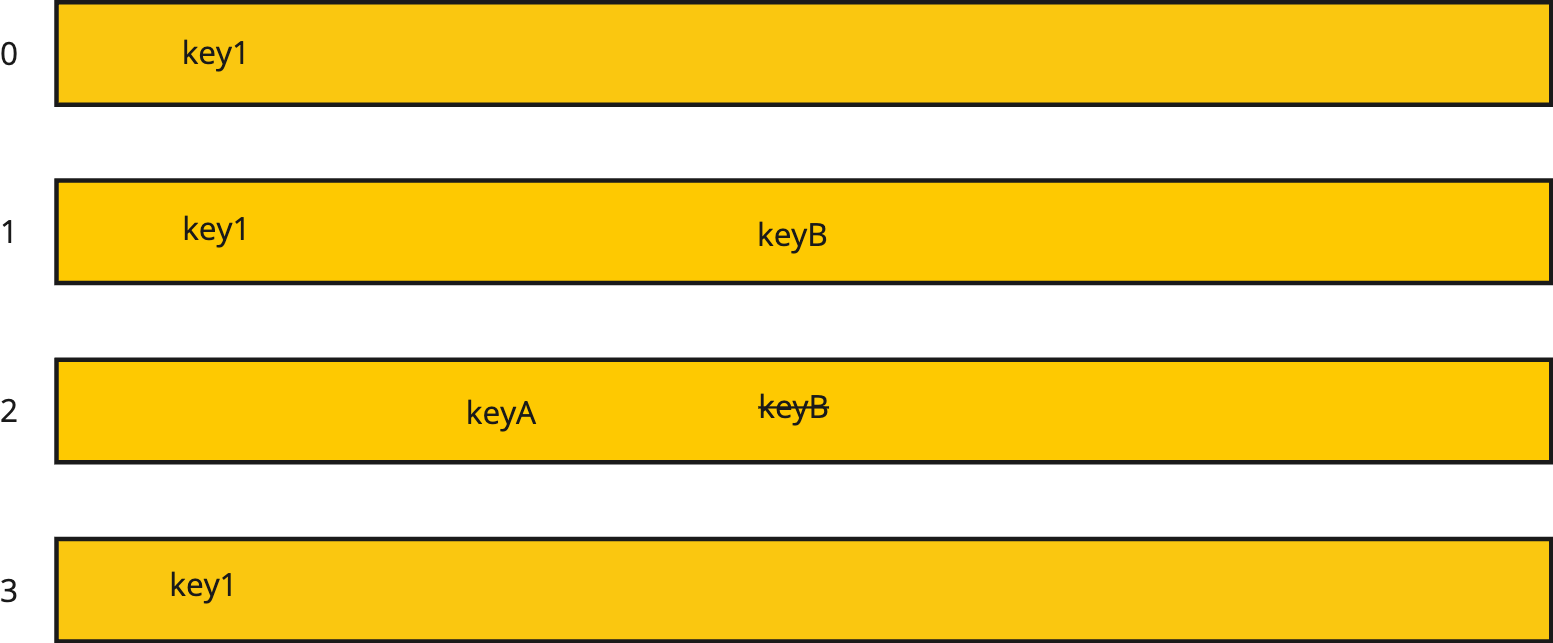
Fig. 28 Working Set
Consider the working set given in Fig. 28 for a dictionary with all persistent keys. The figure shows that checkpoint 0, 1, 2, and 3 are in the working set. During checkpoints 0, 1, and 3 the key key1 was written into the distributed dictionary. During checkpoint 2 a keyA was written into the dictionary. During checkpoint 1 the keyB was written into the dictionary. But during checkpoint 2 keyB was deleted from the dictionary.
Now, if a client comes along that’s got checkpoint 3 as its checkpoint id, and looks up keyB it will not be found. However if another client currently at checkpoint 1 comes along, it will discover keyB in the dictionary. For any key the corresponding value also exists.
The pool can hold duplicates of keys and values. The pool has no restrictions on what can be stored within it. Each dictionary at each checkpoint is a separate dictionary so the keys and values stored at each checkpoint are completely independent of what is stored at other checkpoints.
Assuming that the working set size of this dictionary is 4, then when a new checkpoint comes along it will result in checkpoint 0 being retired. Since key1 exists at checkpoint 2, the key1 from checkpoint 0 is simply deleted from the pool and the dictionary is replaced by a new empty dictionary for checkpoint 4.
Given the current state in Fig. 28 a call to get the length of the dictionary would result in finding 2 keys, key1 and keyA. This can be found by constructing a temporary set of keys. Starting with the keys of checkpoint 0, add all the keys of checkpoint 1, then delete all deleted keys of checkpoint 1. Add in all keys of checkpoint 2 and then delete all deleted keys from the temporary set. Repeat this process for all checkpoint levels in the working set. Then take the length of the computed temporary set and that gives you the length of the dictionary, i.e. the number of keys active at any point in time. Similarly, a call to find all keys at a particular checkpoint level can be found using this algorithm.
Read Operations
Read operations include get operations but also all operations that examine the state of the distributed dictionary. A read operation includes the checkpoint index. Here is how it proceeds:
Client sends get operation to correct manager with checkpoint id, chkpt_id.
If the chckpt_id is older than any checkpoint id in the working set, the oldest checkpoint copy will be examined since that contains the base copy. If wait_for_keys was specified and a reader tries to read a non-persisting key older than the working set, the read is rejected.
If the chckpt_id is newer than any other checkpoint id in the working set, then no worries. We use the newest chkpt_id we have in the working set in that case unless wait_for_keys was specified and this is a non-persisting key. In that case, the reader’s request is queued internally until it can be processed.
Manager receives the message and examines the working_set[checkpoint_map[chkpt_id]] dictionary for the key. If the value is found, great! Return it.
If the key is found in the set of deleted keys for a checkpoint then return that it was not found.
If the key being looked up is not found and the key is a persisting key (i.e. because wait_for_keys was requested), then examine the next older checkpoint in the working set by looking at the checkpoint dictionary and also looking at the deleted_keys set. Keep repeating this until the working set is exhausted or until the key is found. Once the key is found, return its value or return not found depending on where the key was found.
If the key being looked up is not found and is not in the set of persisting keys (i.e. and wait_for_keys was requested) then queue up the request internally until it can be satisfied.
If the working set is exhausted, then report that the key was not found.
For operations where you are given a key, like contains for instance, the algorithm is similar to the one above.
For operations that need global state (like the length operation), you can do set operations to form the correct state information. For instance for length, you would take the union of all the keys in the working set subtracting out the set of deleted keys. This will give you the total length of the dictionary. This is best computed from oldest to newest.
Write Operations
There are two types of write/put operations: one for persistent keys and one for non-persisting keys. When wait_for_keys is True then DDPut is for non-persisting keys, otherwise it stores persisting keys. The DDPPut is the persistent put operation. Exactly what occurs on a put is different for persistent and non-persistent puts.
On persistent puts, steps proceed as follows:
Puts (and deletes) into the distributed dictionary come to a manager. Each put operation now includes a chkpt_id that identifies which checkpoint it is written into. If the chckpt_id does not exist in the working set of the manager, the working set is rotated until it does. Rotating is described in the earlier Retiring and Persisting Checkpoints heading.
A put operation then creates a new entry for the current checkpoint if the key does not already exist in the indicated checkpoint and updates the value if the key already does exist in the current checkpoint.
If the key is deleted it is removed from the checkpoint dictionary if it exists and if the key exists in an older checkpoint, then it is also added to the set of deleted_keys for the checkpoint.
If a put or delete is targeting a checkpoint that no longer exists then the operation is rejected.
For non-persistent puts, the checkpoint id must be in the working set or newer. If it is older than the working set then the put operation is rejected. If it is newer than all checkpoints in the working set, then the oldest checkpoint is examined and if it does not contain all the non-persisting keys of the next newer checkpoint, then the put request is internally queued up for later processing.
Restart
Under certain conditions related to resilience of AI training, it may be necessary to start the distributed dictionary from previous state. In terms of the design of the distributed dictionary, we need to provide a means to start a distributed dictionary from a set of existing memory pools.
To support this, we have a argument, name
(please rename persist_base_name
to
just name
) that can be provided when creating a distributed dictionary. This
name is communicated to the Orchestrator during creation which also distributes
it to each manager as part of the startup process.
The name
argument is used as the base name of the pool created by each manager.
Each manager names its pool as "ddict_"+name+str(manger_id)
. The default
value for name
is None. If None is provided, the orchestrator will randomly
generate a name. This name can be retrieved from the Orchestrator at any point (via
the client) by calling get_name
on the client.
A new argument on dictionary creation, restart
, can be specified along with the
name
argument to specify that the dictionary should be restarted from an
existing memory pool.
There are a few requirements that must be observed for restart to work.
All manager processes must be started on the same nodes where they were started before. If a new node is brought into the mix, the restart on that node will work, but all data associated with that manager will be lost.
There must be the same number of managers started on the same nodes. When restarting a manager it is given the serialized descriptor of its existing memory pool which must already exist on the node where the manager is started.
All managers restart from the state they were in when the dictionary was destroyed with the restart option specified. This will be made clearer in the next section.
Changes to Bringup Sequence (to be edited below and this section to be removed)
As mentioned above, there is one additional argument, restart
, and one renamed
argument, name
. These argument values are communicated with the orchestrator
when the orchestrator is created. They can be passed as part of the pickled
arguments.
When the process group of managers is created, arguments will be specified for
all managers within the process group to provide their manager_id
. Instead of
that being assigned in the DDRegisterManagerResponse, it is now given to the
manager when it starts. In addition, on a restart the serialized descriptor to
the manager’s pool will be provided on the DDRegisterManagerResponse message.
The DDRegisterManager message then likewise changes to include the manager_id
.
Pickling Framework
The general idea, for both manager and orchestrator, is to pickle the state and upon restart the orchestrator and managers recover their state from their pickled states. This is used in the orchestrator to store the map of manager_ids to manager pool serialized descriptors. This same concept is used within the manager to read the pickled state of the manager from a special memory allocation within the pool itself to reconstitute its state.
Making this pickling of state and unpickling work revolves around writing
__getstate__
and _setstate__
methods for several classes. Python classes like
list
, dict
and so forth already have these methods defined so no extra code
is required for them. The constituent items in these data structures do require
these methods. For example, pickling a Python dict
requires that the keys and
values of the dict
also have __getstate__
and __setstate__
methods. So we
have implemented those methods as appropriate on MemoryAlloc
and MemoryPool
.
The __getstate__
of each class returns a serialized descriptor of the object.
The __setstate__
of each attaches to the object represented by the serialized
descriptor.
Destroying a DDict with Restart Option
When destroy is called on a DDict, you can specify that you want restart to be
available. The default value is False
. If allow_restart
is True
then this
is passed to the orchestrator and the orchestrator immediately pickles itself
(i.e. self
) and writes the pickled data to a file named
"/tmp/ddict_orc_"+name
. It then communicates with all managers via the normal
shutdown sequence with the new message field of allow_restart
set to True
.
When the orchestrator finally responds to the destroy back to the client, if
allow_restart=True
is specified, then the name
is returned on the destroy call.
When allow_restart=False
is specified, None
is returned.
(to be deleted eventually) There is a little work to be done to be able to get
the manager destroy completed with restart. In managed_memory.c there is a
function called dragon_memory_alloc_type_blocking
and another called
dragon_memory_alloc_type
that need to be surfaced into the Cython code by
modifying the alloc
and alloc_blocking
methods. Allow a user to specify the
type and type_id themselves if they like on the alloc
and alloc_blocking
calls. If None (the default) is passed, then alloc
and alloc_blocking
should
behave as they do today. Otherwise, use the type and type_id passed in. You can
use the dragon_memory_pool_allocation_exists
to make sure it does not exist
before the allocation is made. If it exists, then the allocation should be
rejected via an exception. Raise an exception of KeyError
in that case.
Each manager, when it receives a destroy message with allow_restart
set to True
,
then calls pickle on itself, which calls __getstate__
on itself. It gets back a
byte array and makes an allocation of type BOOTSTRAP
in its managed memory pool
with a type id of 1 and of the correct size to hold the pickled data.
This will mean writing __getstate__
and __setstate__
for several classes in
the manager file including the WorkingSet
, the Checkpoint
, and the Manager
class itself. Any pending operations are logged as being discarded and discarded
when destroying the dictionary.
The result of pickling the manager is written into the special BOOTSTRAP
allocation and the manager does not destroy the pool when allow_restart
was
specified on the destroy message.
Please note that channels and FLIs are not pickled in either the orchestrator or the managers. FLIs are recreated when the dictionary is restarted and serialized FLIs are communicated as usual during the startup sequence. Clients are not pickled by restarting and must be re-created and attached as normal.
Restarting a DDict
When a DDict is restarted by specifying a name
and restart=True
during
creation, this information is first communicated with the orchestrator which
retrieves the state for the map of manager_ids
to serialized pool descriptors
by opening the file named "/tmp/ddict_orc_"+name
and unpickling the contents. Call
__setstate__
on the Orchestrator with the resulting tuple. It will now have whatever
information was offered up by the call to __getstate__
when it was pickled.
Then, the orchestrator goes through normal startup except that it provides the
serialized pool on the DDictRegisterManagerResponse. Recall that the manager_id
is now provided as an argument with the manager ProcessGroup is started.
The manager, with its manager_id and serialized pool descriptor has what it needs to
attach to its pool and reconstitute its internal objects. Call alloc_from_id
on
the memory pool after attaching to get the BOOTSTRAP
type allocation with id 1
.
Then use that byte string you find there to pass to unpickle to set the state of the
Manager by calling the __setstate__
with the tuple that is returned by unpickling the
data found there.
When setting state in the manager there are checks that can be made. For instance, the working set size must be specified and should be the same as that found in the pickled data.
We don’t blindly set all state in the manager. For instance, we still go through the normal bringup sequence. It’s just that we add the step to reconstitute the working set, checkpoints, and portions of the manager itself. Any state regarding bringup is set as normal so that normal bringup occurs with or without the reconstitution of objects.
In the orchestrator, when setting the state there, the number of managers should be the same as found in the pickled data.
Upon a successful restart (after everything is up and running) the BOOTSTRAP
memory allocation should be freed. This should not be freed until it is clear that all
managers were successfully restarted.
If attaching to the managers memory pool and lookup of the BOOTSTRAP
allocation
is not successful, then we simply do not reconstitute the managers objects and
all keys stored on the manager are lost. In that case a LostKeysError
exception
is raised in the manager to make it clear that manager(s) lost key information.
The message should include the manager ids of the managers that lost keys.
Then the DDict proceeds with the rest of the bringup sequence.
Destroying a DDict without Restart
The default is to destroy the DDict without a restart option. If restart=False
is specified, then in the orchestrator the orchestrator will look for a file
named "/tmp/ddict_orc_"+name
and will delete it during the destroy process.
Cross-dictionary Synchronization
As mentioned above, during restart of the dictionary, we restore the previous state of the distributed dictionaries by re-attaching managers to a set of existing pools from the previous set of nodes. In multi-node training, if any of the previous nodes have drained/crashed and Dragon failed to restart on the node, the managers associated with the drained node are started on new, fresh nodes and will not have access to any previous data. We call these managers empty.
In maching learning it is frequently the case that training is occurring on multiple nodes with exactly the same data. When multiple processes are training using the same data and they each are using a distributed dictionary, the dictionaries are exact copies of each other. We call these dictionaries parallel dictionaries, meaning they are parallel copies of each other.
To reconstitute the data for empty managers, we need full managers with the corresponding manager ID in a parallel distributed dictionary to supply their data to their corresponding empty managers. Full managers are the sources for empty managers to reconstitute their data. The existence of parallel dictionaries make is possible for an empty manager to recover its lost data by retrieving its state from a copy of the manager in another parallel distributed dictionary.
There is a client class method for this purpose. It’s called synchronize_ddicts
,
with the argument serialized_ddicts
which is a list of serialized parallel
distributed dictionaries that should synchronize with each other.
In the function synchronize_ddicts
, the client requests a list of empty
managers and all managers’ serialized main FLIs from the orchestrator of each of
the parallel dictionaries listed in serialized_ddicts
. The client then iterates
through the two lists. For each manager ID, it builds two additional lists: one
containing serialized main FLIs of the empty managers and another containing
serialized main FLIs of the full managers. The client includes the serialized
main FLI of the empty manager in the recovery request and sends it to each
corresponding full manager. This allows the full managers to directly send their
state to the empty manager, enabling multiple empty managers to synchronize in
parallel.
A full manager, upon receiving the request for its state, then pickle itself by
calling __getstate__
on itself. The full manager also builds a mapping of all
memory allocations within its managed memory pool, where the key is a memory ID
and value is the corresponding memory allocation. After gathering all state and
metadata the manager then sends the pickled state, followed by the pairs of
(memory ID, memory allocation) metadata by iterating over the map of its memory
allocations.
After receiving the state from the full manager, the empty manager clears its own
existing data and state, and then deserializes the full manager’s states by
calling __setstate__
to reconstitute the state of itself. The empty manager
continues receiving the metadata pairs until EOF of the stream. Values are stored
temporarily in a map called indirect
where key is the memory ID and value is
the memory allocation in the empty manager’s pool. The empty manager then calls
redirect
on the working set, which calls redirect
on each checkpoint within
the working set. The redirection goes through each set and map of the checkpoint,
rebuilding it by getting the pairs of memory ID and memory from the indirect
map. The full manager receives the response from the empty manager once it has
done all the redirection. Finally, the full manager sends the response to the
client to confirm reconstitution of the empty manager has been completed.
Dictionary Clone
As mentioned in cross-dictionary synchronization, in the resiliency training,
having multiple parallel dicitonary with the same data set allows a dictionary
that loses data to recover data by synchronizing with another parallel
dictionary. To achieve this, users might need to load the same data multiple
times in order to write it to each parallel dictionary. However, loading data
from disk is an expensive operation and should be minimized to improve
performance. The dicitonary API clone
addresses this challenge by cloning a
distributed dictionary to another in memory, thus avoiding redundant disk
operations.
The clone
API performs cross-dictionary synchronization between the source and
destination dictionary. The argument clone_list
represents a list of serialized
destination dictionaries. Clone then clears the data in the destination
dictionaries and recovers the data by cloning memory from the source dictionary
using the the same code path described under cross-dictionary synchronization.
Dictionary Copy
The copy
API serves similar purposes as dictionary clone, except that calling copy
on the dictionary returns a new dictionary containing the same key-value pairs. Note
that calling copy
from the original dictionary is the only valid way to create a
copy, calling copy
from handles is invalid and will result in an exception.
When performing a dictionary copy, a new dictionary is created, and the serialized
descriptor of both the new and the original dictionary is passed as the argument
clone_list
to the dictionary clone operation. Once the clone is complete, the entire
dictionary copy operation is finished.
Custom Pickler for Data Interaction Between C++/Python Clients
Customized picklers are required to enable user to share data through Dragon Distributed
Dictionary between Python and C++ dictionary client. The default pickler used internally
for Python client is cloudpickle
, which serializes keys and values into pickle objects
and send them through FLIs(i.e. File Like Interfaces). The C++ clients,
however, cannot deserialize pickle objects. Therefore, we need to replace the default pickler
with a user-defined pickler that serializes objects into a format that is both serializable
and deserializable by both Python and C++. Additionally, on the C++ client side, users should
implement the serialize
and deserialize
functions in a class that extends from the
Serializable
interface in dragon/dictionary.hpp
. These functions serves the similar
purpose like custom picklers, defining a way to serialize, send, deserialize and receive data
through FLIs. For more details of the Serializable interface and its implementation, refer to
Dragon Distributed Dictionary C Client.
While defining the pickler, users should understand the type and shape of data they will interact with. Given this prerequisite, we can also inlcude any necessary headers with the data and deserialize it them upon receiving.
There are two types of picklers available for Python users to define: the key pickler and value pickler. Key pickler serializes and deserializes key object and interact with FLI, while value pickler serves the same purpose for value.
For the key pickler, two functions must be defined, dumps
and loads
. The dumps
function serializes key object into a bytearray, while the loads
function deserializes
key by reconstructing bytearray into the expected object. Similarly for value pickler,
dump
and load
functions serve the same purpose for value. The example of key and value
picklers are as shown in Listing 58. Additionally, while
deserializing bytes through value pickler, if the expected memory is contiguous and its
size is known already, we can avoid creating extra copies of memory by specifying the
memory chunk size to request as the size of the memory and passing it as an argument while
reading bytes from FLI.
To use the customized pickler, we use the dictionary as a context manager and pass instances of key and value picklers during the initialization. You can provide either key or value pickler, or both. The dictionary automatically switches from default pickler, cloudpickle, to customized pickler for all operations within the context. If no customized pickler is provided, the default pickler is used.
1class numPy2dValuePickler:
2 def __init__(self, shape: tuple, data_type: np.dtype, chunk_size=10):
3 self._shape = shape
4 self._data_type = data_type
5 self._chunk_size = chunk_size
6
7 def dump(self, nparr, file) -> None:
8 mv = memoryview(nparr)
9 bobj = mv.tobytes()
10 for i in range(0, len(bobj), self._chunk_size):
11 file.write(bobj[i : i + self._chunk_size])
12
13 def load(self, file):
14 obj = None
15 try:
16 while True:
17 data = file.read(self._chunk_size)
18 if obj is None:
19 # convert bytes to bytearray
20 view = memoryview(data)
21 obj = bytearray(view)
22 else:
23 obj.extend(data)
24 except EOFError:
25 pass
26
27 ret_arr = np.frombuffer(obj, dtype=self._data_type).reshape(self._shape)
28
29 return ret_arr
30
31class intKeyPickler:
32 def __init__(self, num_bytes=4):
33 self._num_bytes = num_bytes
34
35 def dumps(self, val: int) -> bytearray:
36 return val.to_bytes(self._num_bytes, byteorder=sys.byteorder)
37
38 def loads(self, val: bytearray) -> int:
39 return int.from_bytes(val, byteorder=sys.byteorder)
40
41# To enable reading and writing the same key between C++/Python client, this should be
42# number of bytes for a C++ integer for the customized int key pickler, typically 4,
43# depending on system and compiler.
44INT_NUM_BYTES = 4
45with ddict.pickler(key_pickler=intKeyPickler(INT_NUM_BYTES), value_pickler=numPy2dValuePickler((2, 3), np.double)) as type_dd:
46 # read and write 2D NumPy using customized key and value picklers
47 key = 2048
48 arr = [[0.12, 0.31, 3.4], [4.579, 5.98, 6.54]]
49 value = np.array(arr)
50 type_dd[key] = value
Batch Put
Typically, in AI training, training data is stored in a key-value store like the
Dragon Distributed Dictionary. In the Dragon Distributed Dictionary, each write
operation for a key generates a request from the client and a response from the
manager. When a large amount of training data needs to be written into the
dictionary, this protocal results in a high number of messasges, leading to a
significant communication overhead between clients and managers. To improve
performance and reduce communication time, we introduce the batch_put
API.
Unlike typical put operations, in batch put
, a client only sends a single batch
put request to each manager, regardless of the number of writes it needs to
perform on the manager. Similarly, each manager sends a single response to the
client at the end of batch put.
The batch put operation begins with a call to start_batch_put
and ends with a
call to end_batch_put
as shown in Listing 59. The argument
persist
of the API start_batch_put
is set to true if all keys in the batch
put request are persistent keys. If persist
is set to false then all keys
written during the batch put operation are non-persistent keys. For more details
regarding persistent and non-persistent keys, please see checkpointing in the
Dragon Distributed Dictionary for reference.
1ddict = DDict(1, 1, 3000000, working_set_size=2, wait_for_keys=True)
2
3# writes non-persistent keys using batch put
4ddict.start_batch_put(persist=False) # batch put starts
5ddict["key1"] = "value"
6ddict["key2"] = "value"
7ddict.end_batch_put() # batch put ends
8
9ddict.destroy()
After the batch put operation starts, the client hashes the key and connects to the manager. If the client hasn’t written any keys to the manager during the batch put operation, it will send the first and only batch put request to the manager and keeps the send handle open until the end of the batch put. This allows the client to stream mutilple key-value pairs to the manager throughout the entire batch put operation. The client keeps track of the number of keys written to each manager and also a map of send handles it has created for communiction with managers. At the end of the bath put, the client iterates through the map, closes all send handles and destroys the associated stream channels. Finally, the managers send the batch put responses to the client. The responses include the number of successful writes and manager ID, making it possoible for the client to verify whether all keys are written successfully and report errors if not.
- There are a few notes and constraints for using batch put:
The keys assigned to each worker process must be disjoint.
Proceeding or rolling back a checkpoint during a batch put is not a valid operation.
The persistence of a key during the batch put must align with the argument
persist
specified at the start of the batch put. For example, ifpersist
is set to false, calling the persistent put APIpput
is invalid.
Broadcast Put
During AI training and simulation, one common use case of interacting with a distributed dictionary is when multiple clients request the same model to perform distributed simulations. The model is stored as a single key-value pair in one of the managers within the distributed dictionary. Since the key is hashed and assigned to a single manager, all read requests for the same key from multiple clients are processed sequentially by that manager. This creates a significant scaling pinch point. To improve the scaling performance in this scenario, bput can be used to efficiently distribute a value to all managers of the DDict.
There are two arguments in the broadcast put bput
function, a key and a value.
Writting keys through broadcast put enables the distribution of read requests from
multiple clientsby storing a copy of the non-persistent, key-value pair on each
manager. Since every manager holds a copy of the key-value pair, clients can request
the key from any manager. As a result, multiple read requests for the same key are
distrbuted across multiple managers rather than a single centralized manager.
To efficiently broadcast a key-value pair to every manager, the client selects the
first manager from a randomized list of manager IDs and sends the broadcast put
request message, BPut
, to it. The randomized list of manager IDs is also included
in the request. Similar to a normal put request, and like the Put operation, the key
and value are streamed to the manager. Upon receiving the request, the manager
broadcasts the BPut
request to the first manager on both the left and right halves
of the randomized manager list. It then performs the key-value store operation like
a normal put operation. Each manager responds to the client once it has finished. Note
that when using broadcast with batch put, when using broadcast with batch put, deferred
operations are not supported just as deferred operations are not supported with normal
batch put operations.
To efficiently read the key written through broadcast put, bget (i.e. broadcast get) is used. Unlike normal get operations, the bget operation consults the client’s main manager to look up the value based on its key. The main manager then performs the get operation to read the associated value.
- Notes for using broadcast put:
Calling broadcast put creates duplicates of the key-value pair on all managers
across the distributed dictionary, leading to increased memory overhead. Therefore, users should use this API carefully. Broadcast put and get are desgined to efficiently handle read requests from multiple clients accessing the same key. * While broadcast put can work with multiple clients simultaneously, due to the significant memory overhead as mentioned above, it is recommended to use broadcast put sparingly. It is best used when all clients will need to read the same key-value pair.
Message Flow Between Components
The following sections illustrate the flow of messages between components of the distributed dictionary. All messages are hidden behind the distributed dictionary API. These are internal details of the implementation.
Bringup, Attach, Detach
Creating a distributed dictionary involves a Python client providing information about how many managers, the number of nodes, the total amount of memory, and a policy for where to place managers. The following diagram provides the details of interactions between components for distributed dictionary bringup. Message definitions appear in the aptly named section below. There are a few notes here about this flow.
The client/manager attach flow (see the diagram) is not necessary when a client has sent the DDRegisterClient to a manager. In other words, the DDRegisterClient does all the work of the DDRegisterClientID* message when it is sent to a manager so it does not need to be repeated.
Not pictured in the diagram, the Orchestrator supports the DDRandomManager message and respond to it since some clients may have been started on a node without a manager. When that occurs the client will receive a Not Found response to the SHGetKV message. In that case the client should fall back to sending the DDRandomManager message to the Orchestrator
Each Manager and the Orchestrator are assigned a range of client IDs to assign. The Managers get 100,000 each based on the manager ID and starting at 0. The Orchestrator gets the rest. In this way no two clients will get the same client ID. Necessarily, client IDs will not be sequentially allocated across all nodes.
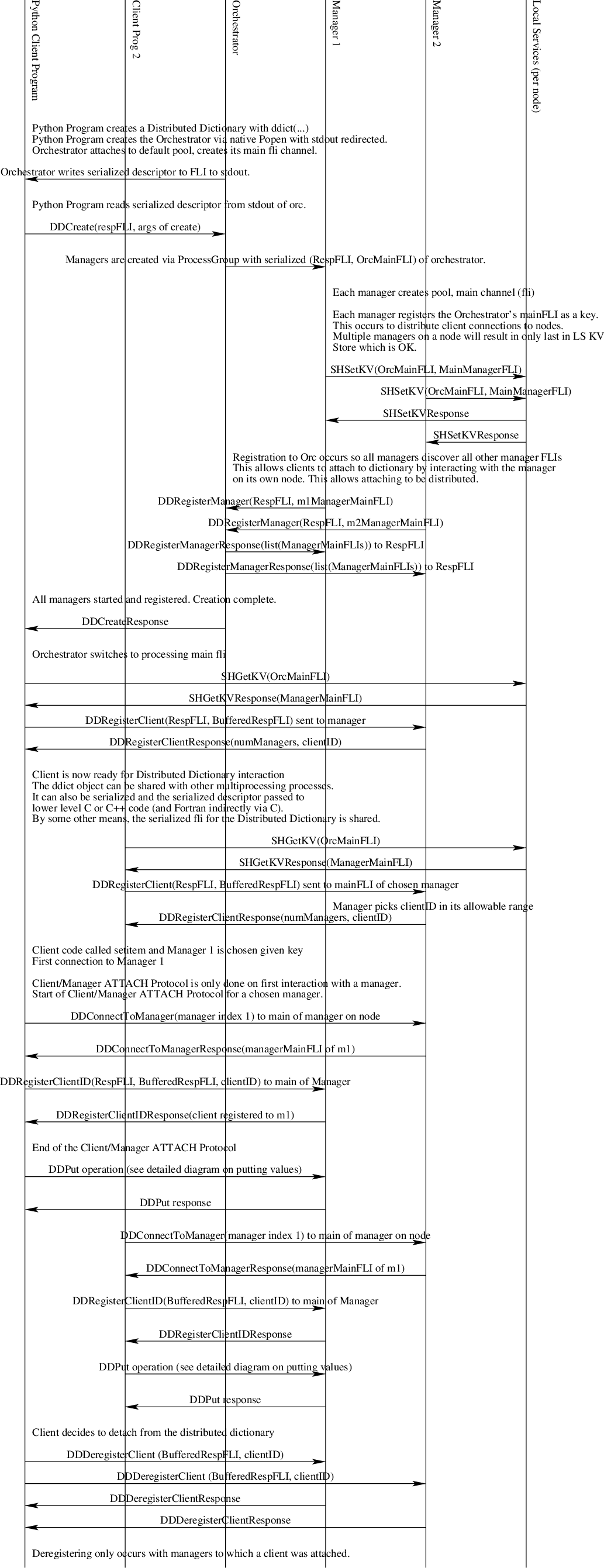
Fig. 29 The Distributed Dictionary Bringup, Attach, Detach Sequence Diagram
Teardown
Bringing down a distributed dictionary is initiated by one client. Other clients should already be aware the dictionary is being destroyed. If not, they will begin to get errors when interacting with the dictionary since channels will no longer exist.
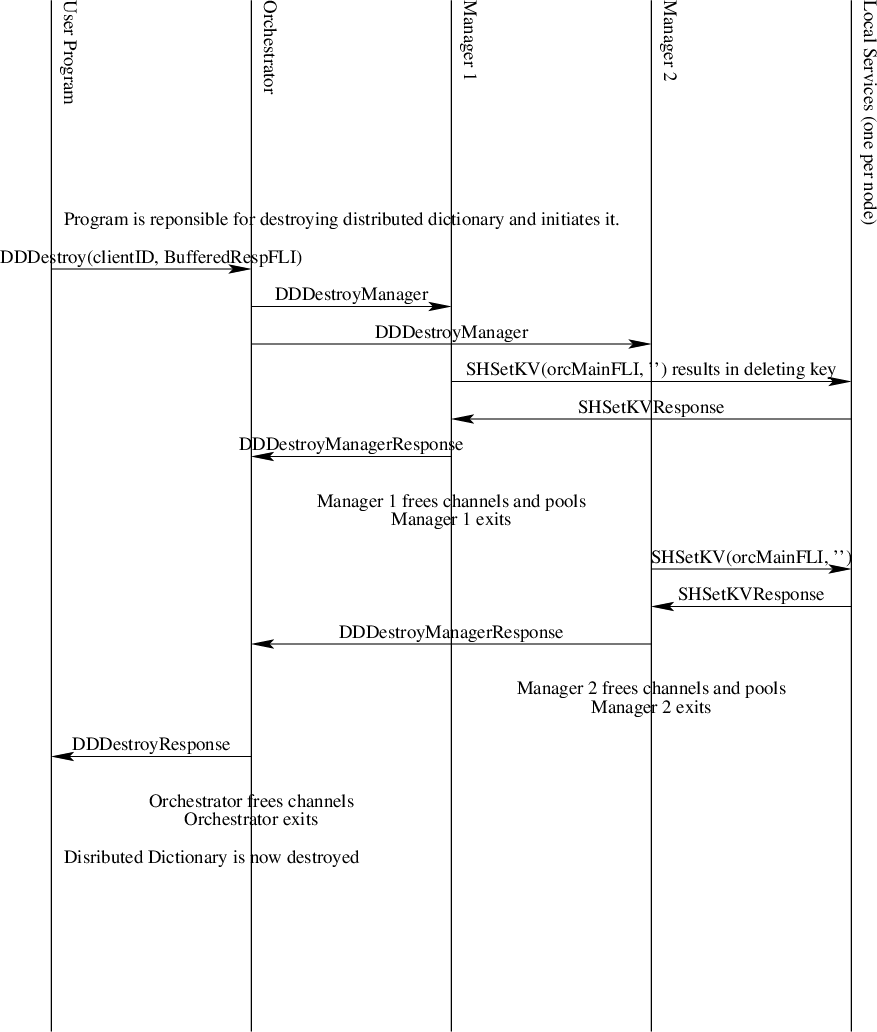
Fig. 30 The Distributed Dictionary Teardown Sequence Diagram
Put and Get Interaction
Puts and gets are initiated by client programs. The key is hashed by the client program’s put or get API call and divided by the number of managers to obtain the integer remainder (modulo operator) value. That value picks which manager is responsible for the put or get operation for the given key. It is imperative that all clients use the same hashing function and that all managers are in the same order for all clients.
Put and get operations are designed to minimize the number of copies of data that are made when they are performed. By having each manager create their own FLI stream channels, keys and values sent to the manager are automatically allocated from the manager’s pool since allocations sent to a channel use the same channel’s pool by default within the dragon api.
Internally to managers they see only managed memory allocations. Each key is one allocation. Values are streamed to the manager through the file-like interface, so values are typically a sequence of managed memory allocations. The internal dictionary of each manager is a map from a managed memory allocation to a list of managed memory allocations.
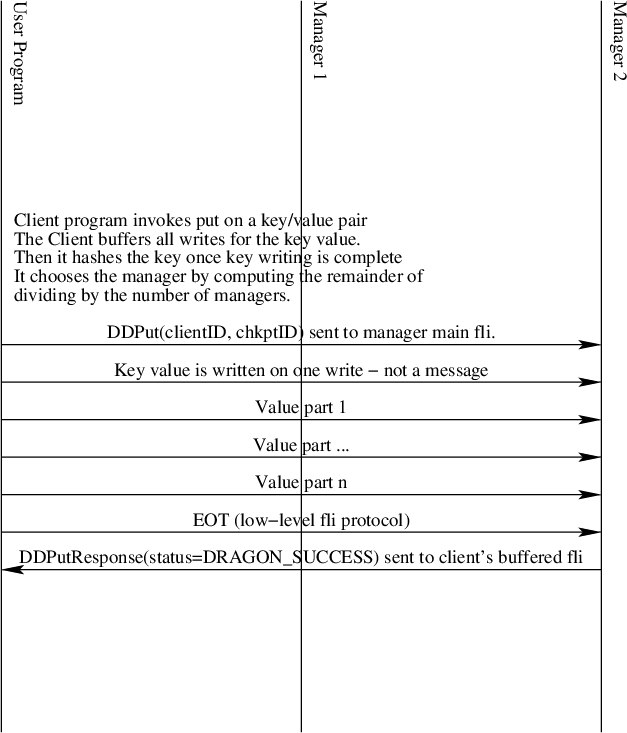
Fig. 31 The Distributed Dictionary Put Sequence Diagram
Likewise, as shown in Fig. 32 the value is streamed back to the client on a get operation. Closing the send handle results in the EOT being transmitted. The client simply reads values for multi-part values until EOT is signaled. In the low-level interface this surfaces as DRAGON_EOT return code. In Python it is signalled by an EOFError exception.
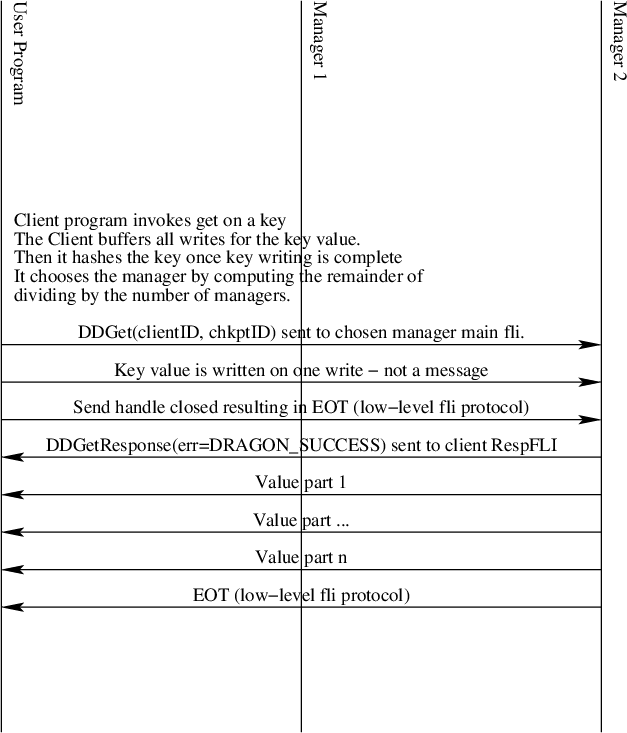
Fig. 32 The Distributed Dictionary Get Sequence Diagram
Pop
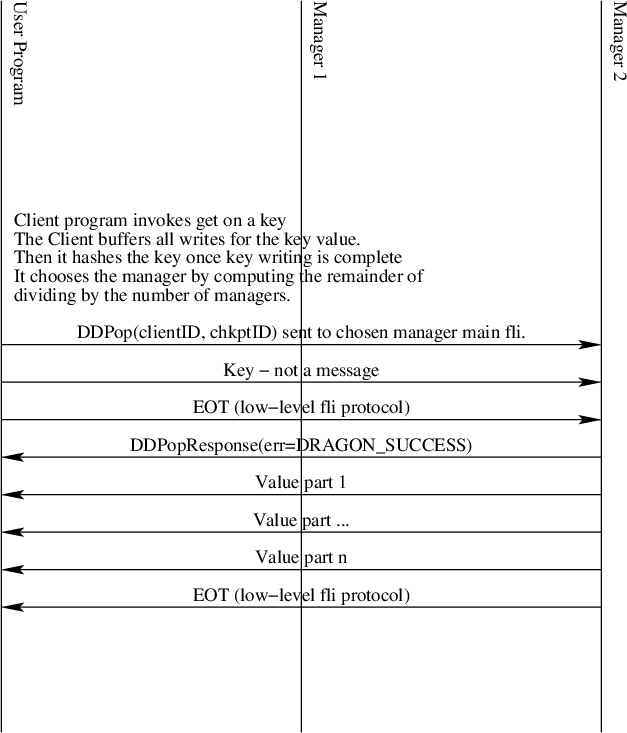
Fig. 33 The Distributed Dictionary Pop Sequence Diagram
Contains
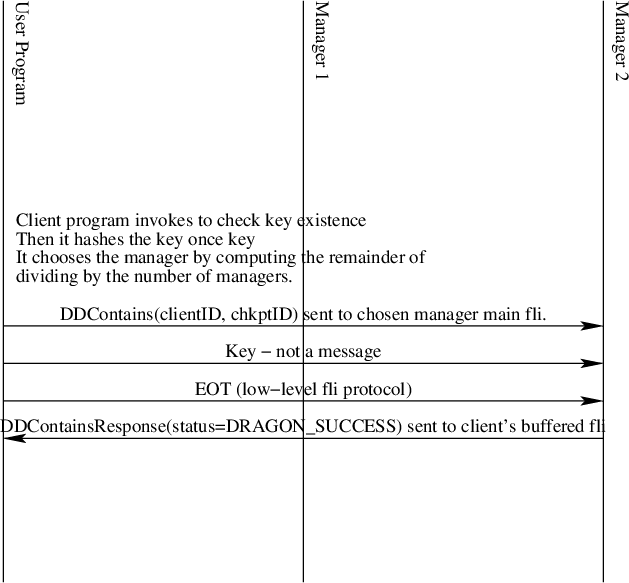
Fig. 34 The Distributed Dictionary Contains Sequence Diagram
Length
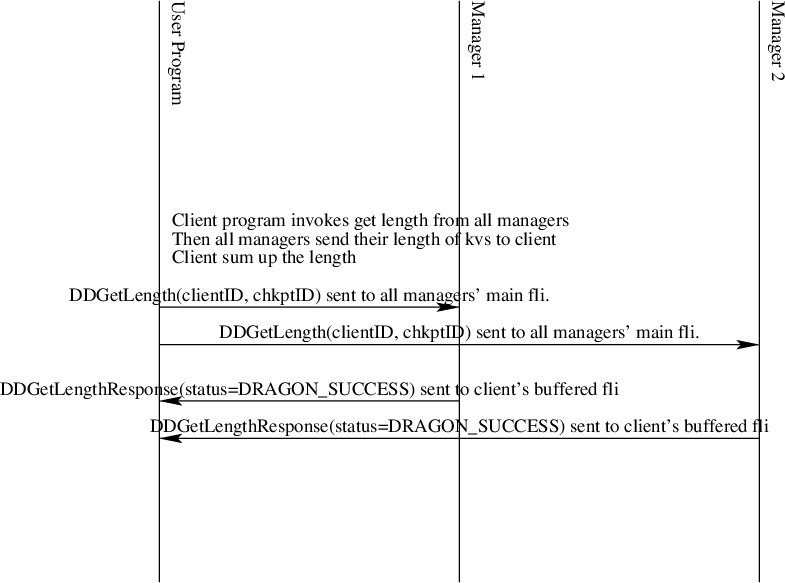
Fig. 35 The Distributed Dictionary Get Length Sequence Diagram
Clear
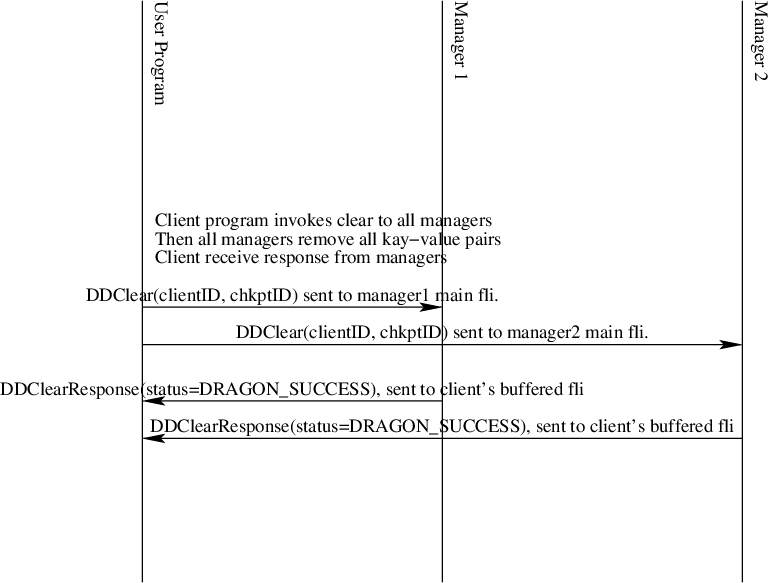
Fig. 36 The Distributed Dictionary Get Length Sequence Diagram
Get All Keys
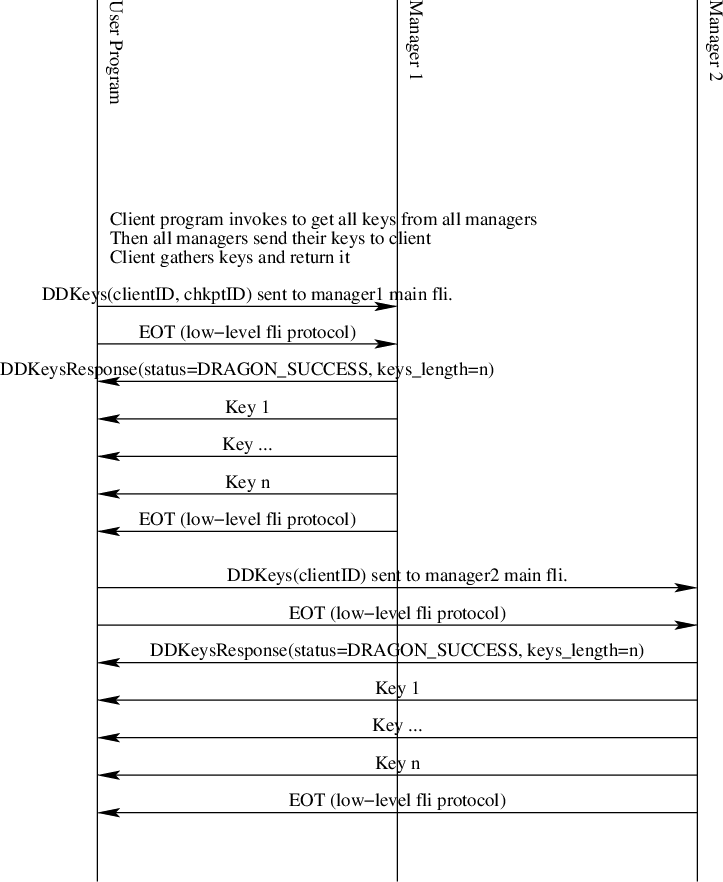
Fig. 37 The Distributed Dictionary Get Length Sequence Diagram